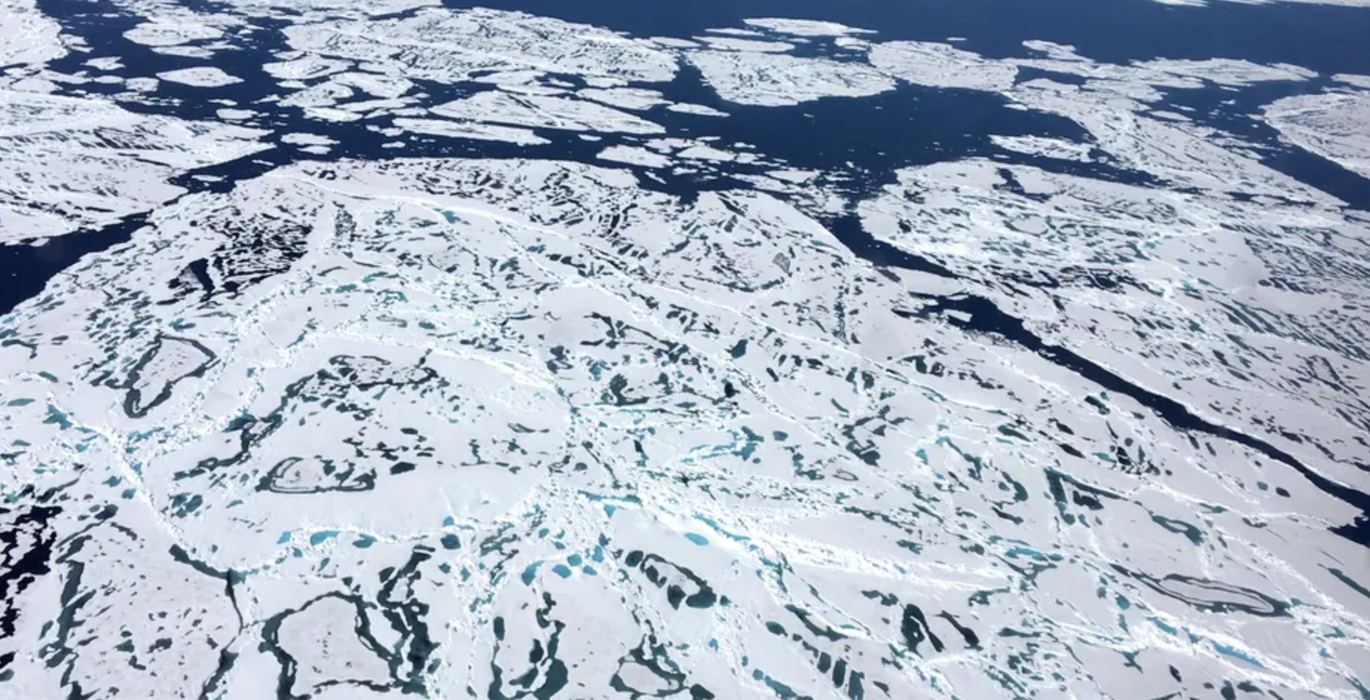
Climate models are an integral part of climate research, especially when studying the Arctic. Models use complex equations and observations to better understand how different aspects of an environment interact with each other. This in turn helps researchers test theories and solutions as well as gain a better understanding of the role each factor plays within a complex system.
With the Arctic changing more rapidly than any other place on Earth, it has become common to see research modelling the effects of these changes into the future. So how do climate models work and why are they important to understanding the regions being studied?
Laura Castro De La Guardia is a postdoctoral researcher at the University of Manitoba and focused her PhD research on climate modelling in Arctic Canada. She answers some of the common questions about climate modelling.
Q: What is climate modelling?
Climate modelling is the modelling of the Earth system’s climate. Through climate modelling a researcher can experiment with the Earth System to determine how the climate will change in the future and identify the responses to that change. So, it is like having planet Earth inside a computer. In the climate models I have used there are different levels of complexity. Complexity can be defined in the form of Earth components (e.g., solid earth, ocean, glaciers, etc.) and resolution.
You could resolve the ocean, the land and trees, have an atmosphere and the sea ice. All these components interact following the physical laws that we understand today from observations. The physical laws are interrelated by equations, and there is a very high number of equations that are connected. The model solves these equations and produces an output, which we analyze to find the answers we are looking for; whereas it is the time of the change, how it happens, or how a specific variable responds to that change.
"To reduce the errors in the model you have to slightly change the equations as you learn more about the physical environment. Also adjusting the parameters depending on the question may help improve the solution."
Q: Why is climate modelling so important in studying climate change?
A: I believe it is very important because it allows us to prepare; instead of a reacting behaviour, we can be proactive.
While climate models are not the exact future, they do give us an understanding of how things could change. To produce a prediction, it is common for scientists to use several simulations and several models to have a range in the response, because each model and simulation will produce a slightly different solution. These solutions can be averaged but could also be presented as of future scenarios. These scenarios are our “likely” future, therefore we can use them to prepare for the change and have options to act proactively instead of waiting for the change to surprise us.
Q: What are three types of climate models and how do they differ?
A: The three most common types of climate models are the general circulation models (GCMs), the intermediate complexity models and the energy balance models (EBMs).
I have only worked directly with the general circulation models. These are the most complex models and they are also considered the most accurate. The GCMs can have multiple compartments such as, ocean, land, atmosphere, cryosphere, biosphere and chemistry.
They account for thermodynamical and dynamical processes within each compartment and the interactions and exchanges between compartment (e.g., river entering the ocean, the role of the biosphere on atmospheric CO2).
The output is 3D (longitude, latitude and depth) with the number of grid cell depending on the resolution. There are a number different GCMs available. More often than not, a climate prediction is made by running several GCMS simultaneously. This allows the scientists to have a mean solution as well as a range of future scenarios from which to estimate an uncertainty. For example, while all GCMs predict sea ice will be lost from the Arctic in summer, the timing of this event differs between models.
Energy balance models (EBMs) are simpler. They are focus on the Earth’s energy budget and consider only thermodynamic processes (e.g., heat radiation entering and leaving Earth). These models make no attempt to resolve the dynamic components of the Earth (e.g., atmosphere and ocean circulation). They are typically used to predict long-term changes of the Earth’s temperature as one value averaged over the entire globe. They can also be used for regional scale, but one model predicts only one region.
I am not as familiar with the intermediate complexity models, but my understanding is that they are in between the GCMs and EBMs.
This video explaining climate modeling was created by the Commonwealth Scientific and Industrial Research Organisation (CSIRO) in collaboration with Australian Bureau of Meteorology.
Q: What is a typical margin of error and what do you do to reduce that?
A: To reduce the errors in the model you have to slightly change the equations as you learn more about the physical environment. Also adjusting the parameters depending on the question may help improve the solution.
Parameters are constants that represent a process that the model cannot resolve either because of the model resolution or because we do not understand it well. However, we can only improve the solution based on the past, because that is when we have observations. So, we always use the past observations to evaluate our models and that is why most of the parameters are adjusted to the past environment. Ultimately the future simulations are based on how well we reproduce the past.
So, if I give you the example of the sea ice, you can have a past observation of sea ice decline and then you can have the model prediction of the sea ice decline. These predictions are always off, the model rate of decline is always slower than the observation.
Modellers recognize that this discrepancy between the observations and the model solution could be the result of missing feedback in the model that doesn't allow for the sea ice to melt as fast as it is actually melting in the observations.
So, when we looked at the model projections into the future, we need to look to them with a conservative eye. It is more advisable to work with a range.
Additionally, in some cases, increasing the model resolution can also help improve the model results. At higher resolutions more processes can be resolved instead of being parameterize.
Q: How does resolution factor into a model?
Resolution is very important. Increasing resolution can significantly improve the climate model results. One reason is that at higher resolution a model can resolved the smaller scale processes that otherwise needed to be parametrized.
The classic example of this is the representations of the eddies in the fluid. However, by increasing resolution the computational requirements and the amount of space to store the output are significantly higher, especially when considering that most climate simulations are commonly run for 100s of years. Hence the scientist must balance computational requirements and accuracy, which are usually defined by the project goals.
Q: The terms weather and climate are sometimes used interchangeably. How do weather models and climate models differ?
Weather refers to short-term atmospheric conditions whereas climate is the averaged weather over a long period. This distinction can also be extended to the difference between the two types of models.
Weather models predict/forecast over short timescales and not too far in the future; for example tomorrow or a week from now. They also make the predictions over a relatively small geographical area and usually have very high spatial and temporal resolution. The weather model also uses the most current weather data available and this makes the predictions very accurate.
A climate model is based on the principle of a weather model, but it focuses on longer timescales. Climate models consider additional earth system processes that are not considered in weather models. One such processes is the rate of melting of Greenland glacier, which does not influence the weather (or a short temporal scale) but can have a significant impact on the climate (or a long timescale).
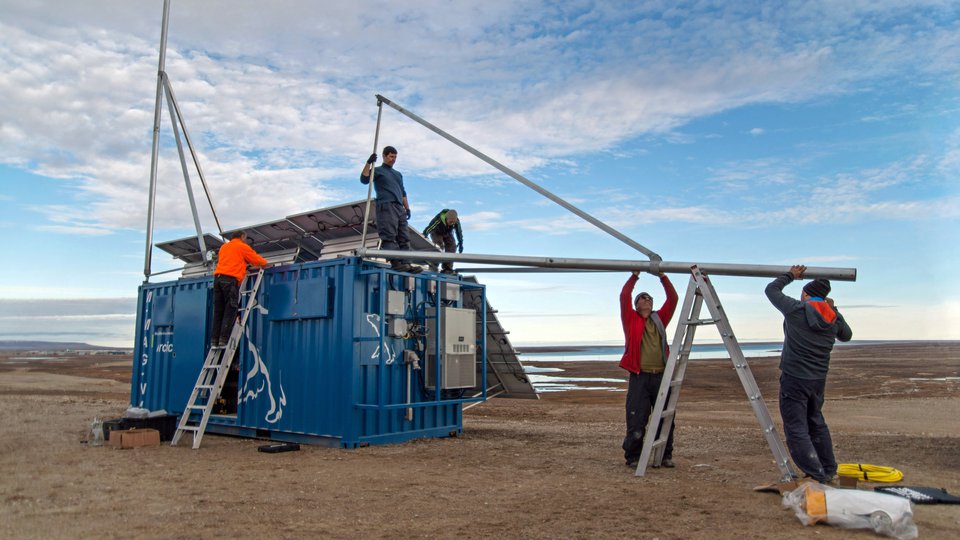
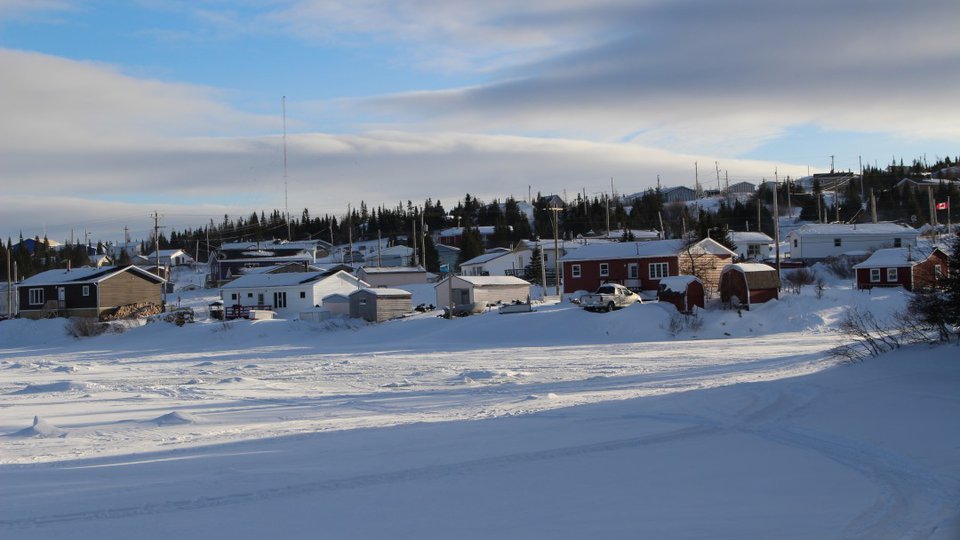
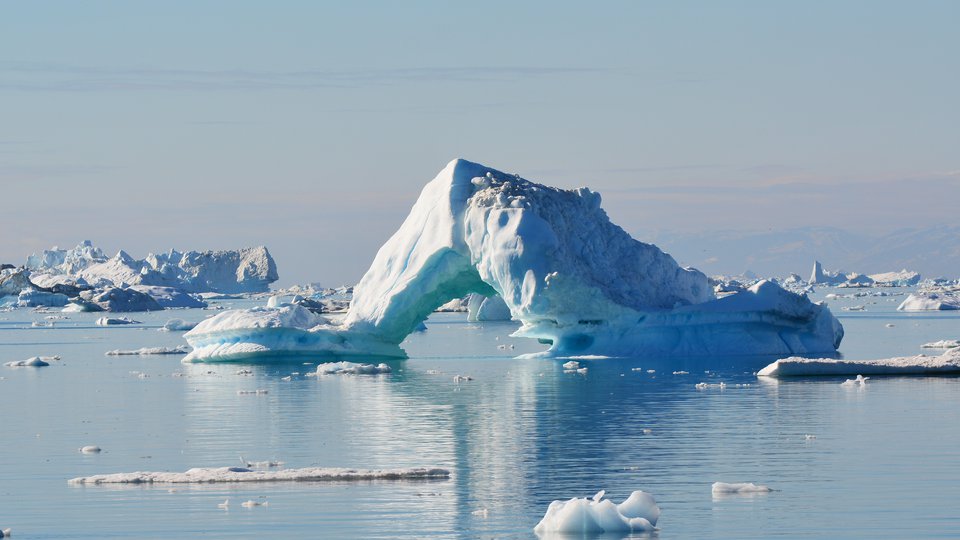
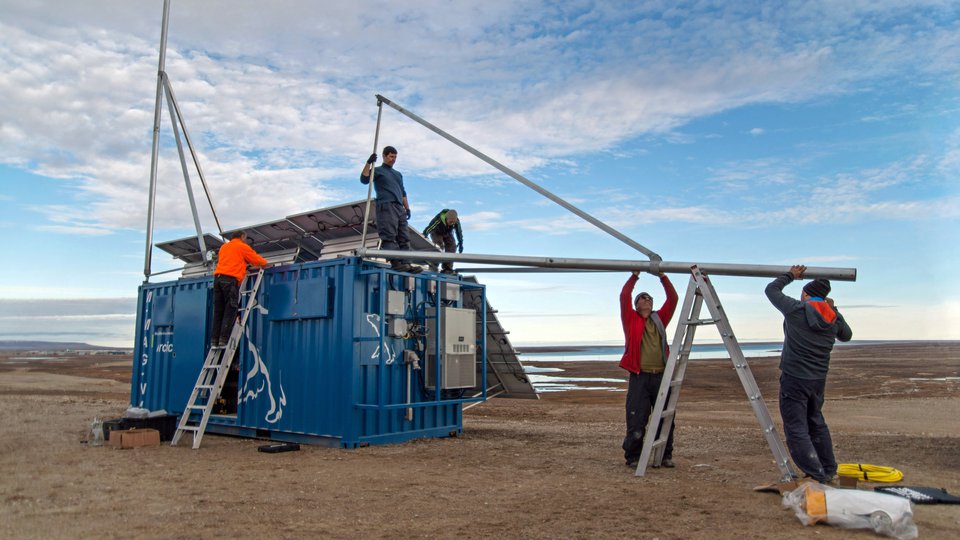
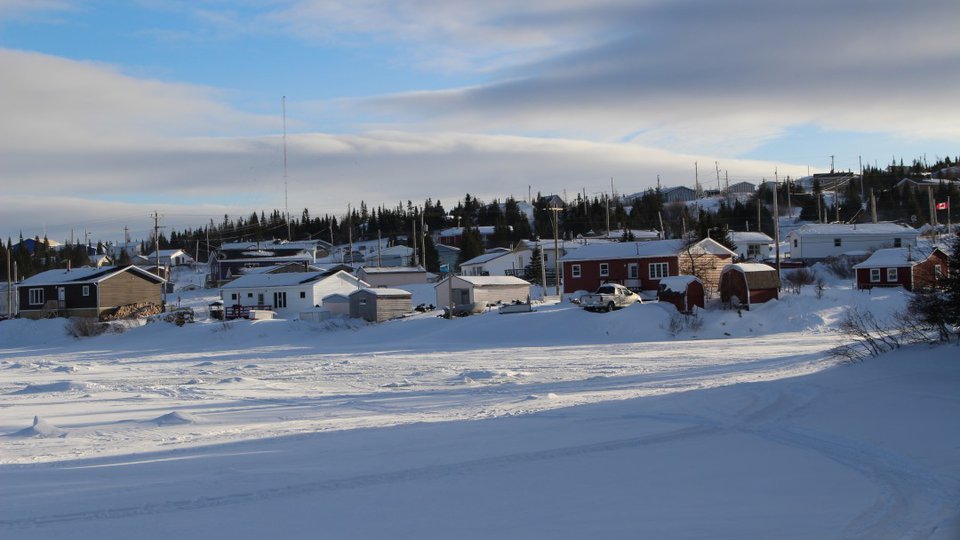
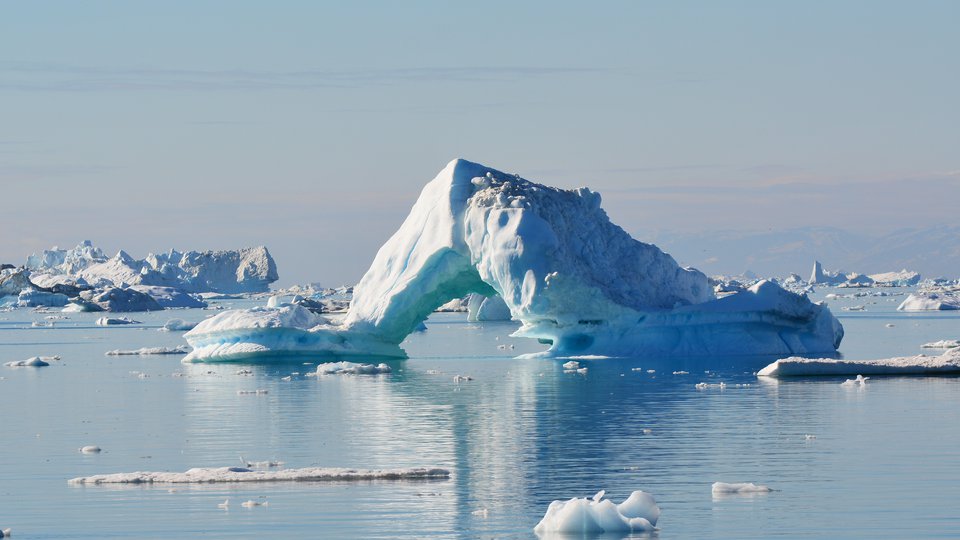